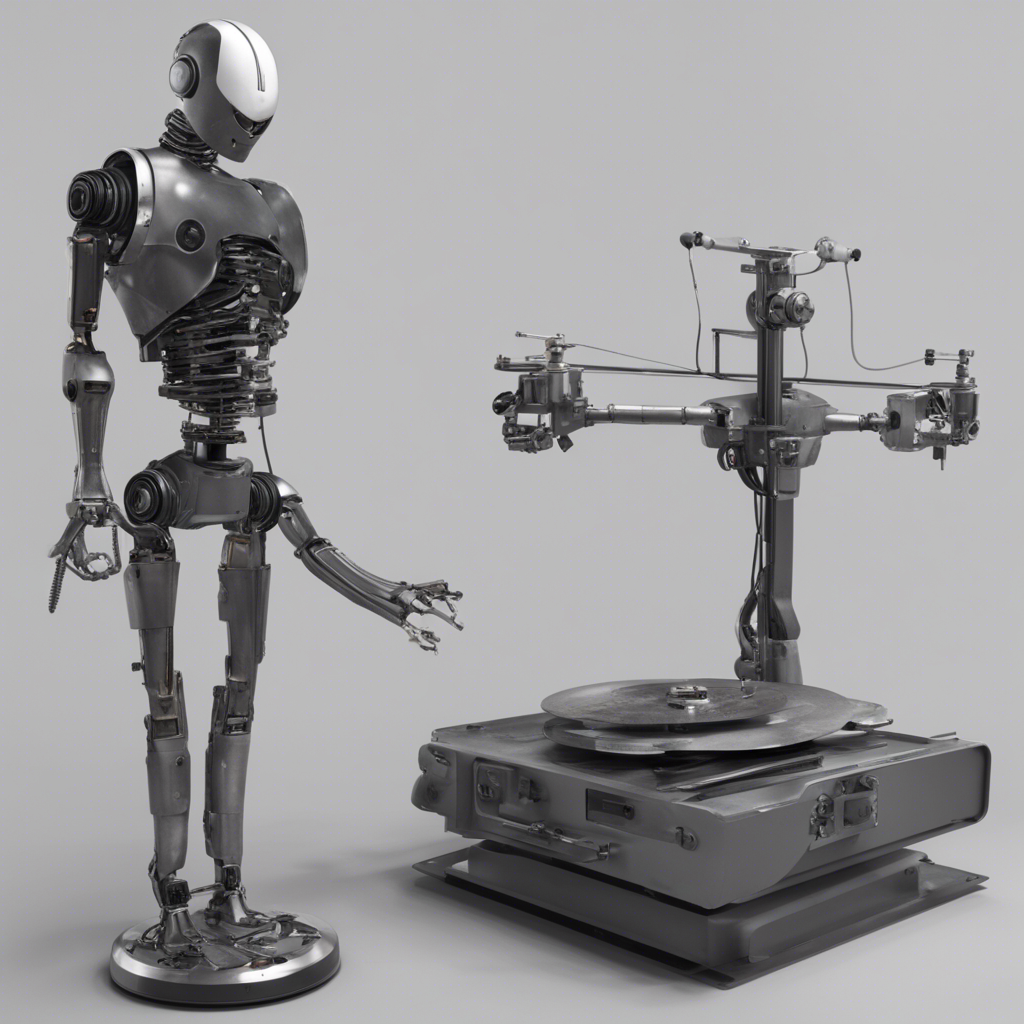
Understanding the Ethics of AI Bias and Fairness
In recent years, the field of Artificial Intelligence (AI) has seen remarkable advancements that have the potential to revolutionize various industries. However, as AI becomes increasingly prevalent in our lives, concerns around bias and fairness have emerged. The ethical implications of AI bias and fairness raise important questions about the potential harm caused by biased decision-making algorithms. In this blog post, we will explore the concept of AI bias, the importance of fairness in AI systems, the challenges in achieving fairness, and the steps being taken to address this issue.
What is AI Bias?
AI bias refers to the phenomenon where machine learning algorithms systematically produce results that are unfair or discriminatory to certain individuals or groups. This bias can occur in various stages of the AI development pipeline, including data collection, data preprocessing, algorithm design, and model evaluation.
The main reason behind AI bias is that machine learning models learn and make predictions based on patterns and trends within the training data they are fed. If the training data contains biased or incomplete information, the resulting AI system can inadvertently perpetuate and amplify this bias.
The Importance of Fairness in AI Systems
Fairness is a fundamental ethical principle that should guide the development and deployment of AI systems. Ensuring fairness means treating everyone impartially and without discrimination. Discrimination can occur based on protected attributes such as race, gender, age, or sexual orientation, as well as other characteristics like socioeconomic status or geographic location.
Unfair AI systems can have far-reaching consequences. For example, biased algorithms used in hiring processes could perpetuate gender or racial inequalities, leading to unequal opportunities for marginalized groups. Biased algorithms in criminal justice systems may result in unfair sentencing or profiling. It is crucial to address these issues to prevent further exacerbation of social inequities.
Challenges in Achieving Fairness
Achieving fairness in AI systems is not a straightforward task. Several challenges contribute to the complexity of this issue:
1. Data Bias:
AI systems are trained on historical data, which may reflect biases present in society. If the training data is biased, the resulting algorithm can learn and perpetuate these biases. Additionally, biased data collection methods or sampling techniques can further exacerbate the problem.
2. Algorithmic Bias:
Even without biased data, the algorithms themselves may introduce bias. Algorithmic bias can arise if the model’s structure or the features it focuses on are inadvertently discriminatory. For instance, if a predictive model assigns higher risk scores to individuals based on their race, it can create biased outcomes.
3. Interpreting Fairness:
Defining and measuring fairness is a complex task. Different notions of fairness may conflict with each other, making it challenging to find a universally acceptable definition. Researchers and practitioners are developing various fairness metrics and methodologies to tackle this challenge effectively.
4. Trade-Offs and Contextual Considerations:
Striving for fairness may involve trade-offs with other desirable properties of AI systems, such as accuracy or efficiency. Balancing these considerations becomes even more difficult when fairness needs to be tailored to specific contexts or protected attributes.
Addressing Bias and Promoting Fairness
To ensure AI systems are fair and unbiased, researchers, policymakers, and industry leaders have initiated several measures:
1. Diverse and Representative Data:
Addressing data bias requires diverse and representative datasets. Efforts are being made to collect data that accounts for different demographic groups, as well as considering the potential biases associated with specific domains.
2. Transparency and Explainability:
Increasing transparency and explainability of AI systems can help identify and understand biases. Techniques like algorithmic auditing and model interpretability provide insights into how decisions are made, enabling the detection and mitigation of biases.
3. Algorithmic Fairness:
Researchers are actively working on developing algorithms that are explicitly designed to mitigate bias and promote fairness. These algorithms aim to strike a balance between accuracy and fairness, considering different fairness metrics and notions.
4. Regulation and Policy:
Policymakers are recognizing the critical importance of addressing AI bias and fairness. Regulatory frameworks are being developed to encourage transparency, accountability, and fairness in AI systems. These frameworks promote the responsible and ethical deployment of AI technologies.
Conclusion
The ethical implications of AI bias and fairness are increasingly gaining attention, as they have the potential to shape the lives of individuals and impact society as a whole. Understanding and addressing AI bias is crucial for building reliable, transparent, and fair AI systems. By considering diverse and representative data, promoting transparency and explainability, developing algorithms explicitly addressing fairness, and implementing appropriate regulations, stakeholders can collectively work towards creating AI systems that are unbiased, equitable, and aligned with our ethical standards.
References:
-
Buolamwini, J., & Gebru, T. (2018). Gender shades: Intersectional accuracy disparities in commercial gender classification. Proceedings of the 1st Conference on Fairness, Accountability, and Transparency, 77-91.
-
Mitchell, M., Wu, S., Zaldivar, A., Barnes, P., Vasserman, L., Hutchinson, B., … & Gebru, T. (2019). Model cards for model reporting. Proceedings of the conference on fairness, accountability, and transparency, 220-229.
-
Barocas, S., & Selbst, A. (2016). Big data’s disparate impact. California law review, 104(3), 671-732.
-
Raji, I. D., & Buolamwini, J. (2019). Actionable auditing: Investigating the impact of publicly naming biased performance results of commercial AI products. Proceedings of the conference on fairness, accountability, and transparency, 62-70.