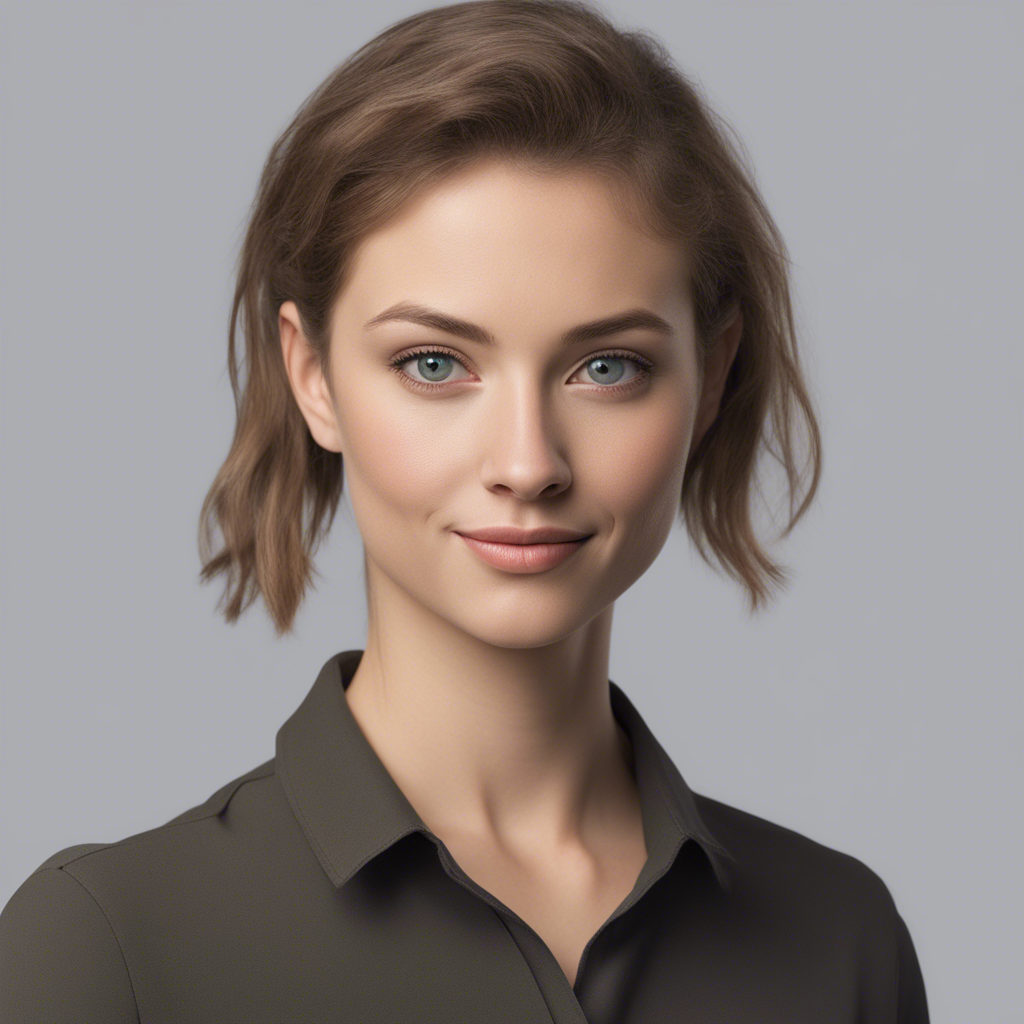
The Ethics of AI Bias: Navigating the Ethical Challenges
Artificial intelligence (AI) has rapidly evolved in recent years, bringing exciting advancements and transformative changes to various industries. From healthcare to finance and transportation to entertainment, AI algorithms now permeate many aspects of our daily lives. However, as we embrace these technological innovations, it is crucial to consider the ethical implications, particularly in relation to AI bias.
AI bias refers to the presence of unfair and discriminatory practices in AI systems that can result in unjust outcomes. These biases can be unintentional, arising from the data used to train AI models, or they can stem from conscious decisions made by developers and organizations. In either case, AI bias can perpetuate and reinforce existing societal inequalities and prejudices.
Understanding AI Bias
To comprehend the nuances of AI bias, we must first understand how AI algorithms are developed and trained. AI systems learn patterns and make decisions based on the data provided to them. Oftentimes, this data is collected from historical records or human interactions. If the training data is biased, the AI system may generate biased results. For example, if a hiring AI algorithm is trained using historical data that is biased towards hiring predominantly male candidates, the algorithm may perpetuate gender bias by favoring male applicants.
Moreover, bias can also occur due to algorithmic design decisions. Developers establish the rules and objectives for AI systems, and any inherent biases they possess can affect the outcomes. For instance, a facial recognition algorithm may be more accurate in identifying individuals with lighter skin tones, leading to misidentifications or exclusion of individuals with darker skin tones.
The Impact of AI Bias
The consequences of AI bias are far-reaching and can impact individuals and communities in various ways. Biased AI systems can perpetuate discrimination based on race, gender, age, or other protected attributes. This leads to unfair treatment in areas such as employment, lending, criminal justice, and healthcare. Marginalized communities often bear the brunt of biased AI systems, exacerbating existing inequalities.
For instance, in criminal justice, AI algorithms used for predictive policing have been found to disproportionately target minority communities. Similarly, biased credit scoring algorithms can discriminate against vulnerable populations, preventing access to financial opportunities. These examples highlight the urgency of addressing AI bias and upholding ethical standards in AI development.
Legal and Ethical Frameworks
Recognizing the significance of addressing AI bias, several legal and ethical frameworks have emerged to guide developers and organizations in navigating this complex landscape. These frameworks aim to identify, mitigate, and prevent biases in AI systems, ensuring they align with ethical standards and legal requirements.
The European Commission’s General Data Protection Regulation (GDPR) sets guidelines for data protection, privacy, and algorithmic accountability, emphasizing the need for transparency and fairness in AI systems. Similarly, the Institute of Electrical and Electronics Engineers (IEEE) has released the Ethically Aligned Design framework, providing tangible guidelines for developers to integrate ethical considerations into the design process. These frameworks encourage responsible AI development and underline the importance of addressing biases.
Strategies to Mitigate AI Bias
Developing unbiased AI systems is a multifaceted challenge that requires a combination of technical, social, and ethical approaches. Here are some strategies that can help mitigate AI bias:
-
Diverse and Representative Data: Ensuring that training datasets are diverse and representative of the population is crucial. Steps should be taken to identify and mitigate any inherent biases in data collection to minimize the risk of biased outcomes.
-
Regular Audits and Monitoring: Continuous evaluation and audits of AI algorithms can help uncover biases and identify areas for improvement. Monitoring the outcomes of AI systems is crucial to detect and rectify any discriminatory patterns that may arise over time.
-
Interdisciplinary Collaboration: Developing unbiased AI systems requires collaboration among diverse teams comprising data scientists, ethicists, sociologists, and domain experts. Incorporating multiple perspectives can help identify and address biases more effectively.
-
Explainability and Transparency: AI systems should be designed to provide understandable and interpretable explanations for their decisions. Transparency around the algorithmic processes helps stakeholders assess potential biases and challenges the black box nature often associated with AI.
-
Stakeholder Engagement: Engaging with various stakeholders, including affected communities, civil society organizations, and regulators, can foster dialogue and enhance accountability in AI development. Involving diverse perspectives can uncover biases that might have otherwise gone unnoticed.
The Future of Ethical AI
As AI continues to advance, prioritizing ethics in AI development becomes increasingly important. Striving for unbiased AI systems is not only ethically imperative but also crucial for long-term viability and trust in these technologies. Continued research, collaboration, and regulation will be imperative to ensure that AI systems are developed and deployed in a responsible and ethical manner.
By acknowledging and actively addressing AI bias, we can move towards a future where AI technologies are fair, transparent, and beneficial for all members of society.
Please note: The information presented in this blog post is intended for informational purposes only and should not be considered as legal or professional advice.
References:
- Buolamwini, J., & Gebru, T. (2018). Gender shades: Intersectional accuracy disparities in commercial gender classification. Proceedings of the 1st Conference on Fairness, Accountability, and Transparency, 77–91.
- European Commission. (2018). Ethics guidelines for trustworthy AI. Retrieved from https://ec.europa.eu/digital-single-market/en/news/ethics-guidelines-trustworthy-ai
- IEEE. (2021). Ethically Aligned Design. Retrieved from https://standards.ieee.org/standard/7000-series-ieee-ethically-aligned-design.html
- Obermeyer, Z., Powers, B., Vogeli, C., & Mullainathan, S. (2019). Dissecting racial bias in an algorithm used to manage the health of populations. Science, 366(6464), 447-453.