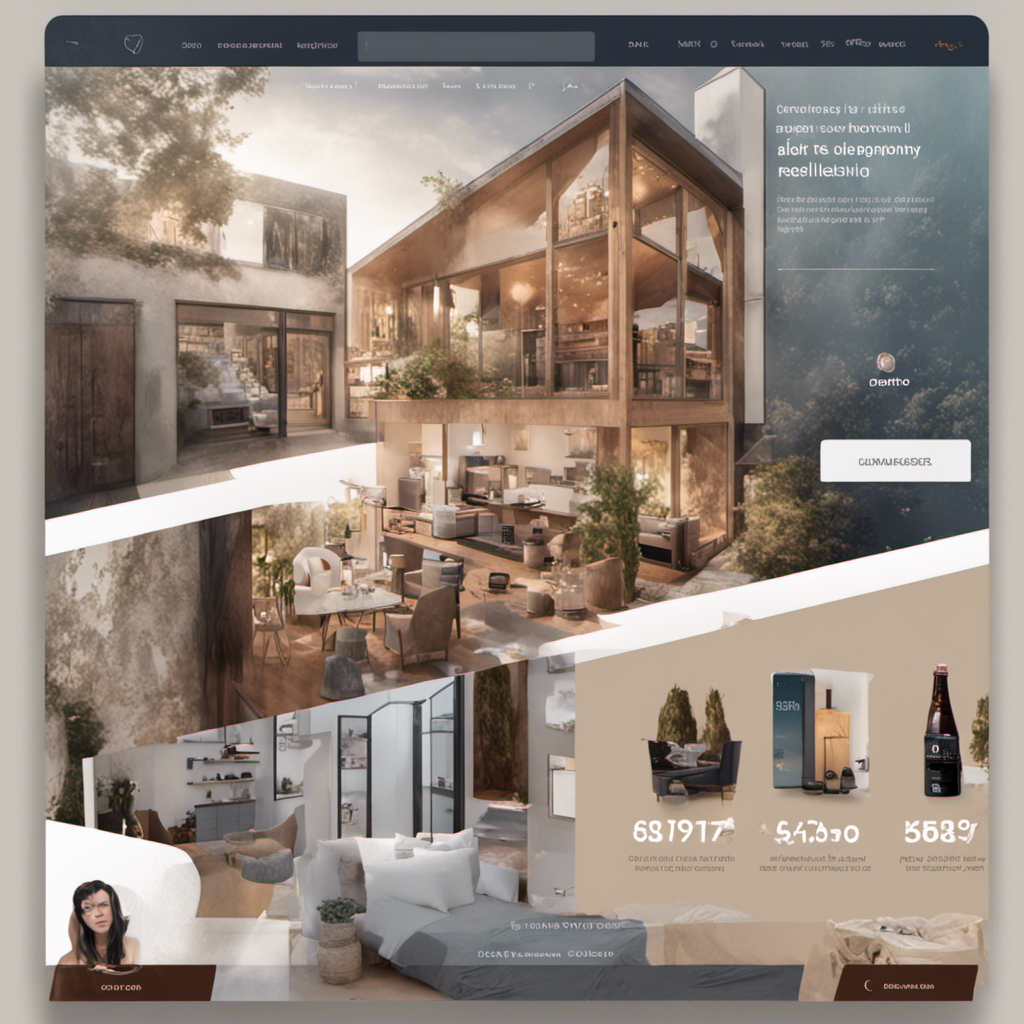
Implementing Machine Learning in E-commerce
Machine learning is a rapidly emerging field that has the potential to revolutionize various industries, and e-commerce is no exception. With the advent of cutting-edge technology and the availability of vast amounts of data, e-commerce businesses can now leverage machine learning algorithms to gain valuable insights and improve their overall operations.
In this blog post, we will explore the various ways in which machine learning techniques can be implemented in the e-commerce industry. We will delve into the specific applications of machine learning, discuss the benefits it offers to businesses, and provide examples of companies successfully utilizing machine learning in their e-commerce strategies.
Understanding Machine Learning in E-commerce
Before we delve into the applications of machine learning, it’s essential to have a clear understanding of what machine learning actually is. Machine learning is a subset of artificial intelligence (AI) that involves the development of algorithms that enable computers to learn from and make predictions or decisions based on data without being explicitly programmed.
In the context of e-commerce, machine learning algorithms can be trained on large datasets to identify patterns, recognize customer behavior, optimize pricing, personalize recommendations, and enhance fraud detection, among other tasks. By leveraging machine learning, e-commerce businesses can gain valuable insights and improve their decision-making processes.
Applications of Machine Learning in E-commerce
-
Product Recommendations: One of the most prominent uses of machine learning in e-commerce is in providing personalized product recommendations to customers. By analyzing customer browsing and purchase history, as well as other relevant data such as demographics and product attributes, machine learning algorithms can suggest products that are most likely to be of interest to a specific customer. This not only enhances the customer experience but also drives sales and customer loyalty.
-
Demand Forecasting: Machine learning algorithms can analyze historical sales data, external factors such as weather conditions or promotional events, and other relevant variables to predict future demand accurately. By leveraging these forecasting insights, e-commerce businesses can optimize inventory management, pricing strategies, and production planning, ultimately minimizing costs and maximizing revenue.
-
Customer Segmentation: Machine learning algorithms can divide customers into distinct groups based on their behavior, preferences, and demographics. This enables e-commerce businesses to tailor their marketing strategies, campaigns, and promotions to specific customer segments, leading to higher conversion rates and customer satisfaction.
-
Fraud Detection: Fraudulent activities pose a significant challenge for e-commerce businesses. Machine learning algorithms can analyze vast amounts of data, including purchase patterns, user behavior, and historical fraud data, to detect and prevent fraudulent transactions in real-time. This helps protect both the e-commerce business and its customers, ensuring secure transactions.
-
Optimized Pricing: Machine learning algorithms can analyze market trends, competitor pricing, customer preferences, and other relevant factors to optimize pricing strategies in real-time. By dynamically adjusting prices based on demand and market conditions, e-commerce businesses can increase their competitiveness, maximize revenue, and improve customer satisfaction.
Examples of Machine Learning in E-commerce
-
Amazon: Amazon is a prime example of a company successfully implementing machine learning in its e-commerce operations. The company utilizes machine learning algorithms to power its recommendation engine, optimizing product suggestions based on individual customer preferences and behavior. This has significantly contributed to Amazon’s success and customer retention.
-
Netflix: While primarily a video-streaming platform, Netflix also incorporates machine learning algorithms to provide personalized recommendations to its customers. By analyzing viewing history, genre preferences, and other relevant data, Netflix effectively suggests movies and shows tailored to each individual user’s taste.
-
Alibaba: As one of the largest e-commerce platforms globally, Alibaba leverages machine learning algorithms to enhance its fraud detection capabilities. By analyzing a vast amount of data, including user behavior, transaction patterns, and historical fraud instances, Alibaba’s algorithms can accurately identify and prevent fraudulent activities on its platform, ensuring a secure and trustworthy online marketplace for its users.
Conclusion
Machine learning has the potential to transform the e-commerce landscape by enhancing customer experiences, optimizing operations, and improving decision-making processes. From personalized recommendations and optimized pricing to demand forecasting and fraud detection, the applications of machine learning in e-commerce are abundant.
As demonstrated by the examples of Amazon, Netflix, and Alibaba, implementing machine learning in e-commerce can lead to significant benefits and competitive advantages. By leveraging the power of data and intelligent algorithms, businesses can better understand their customers, make informed decisions, and ultimately drive growth in the ever-evolving e-commerce industry.
References:
- S. Chawla, D. Bell, J. McGregor, and R. Agrawal, “E-commerce Recommendation Applications: Data Driven Intelligent Interfaces and Internet-Scale Data Processing”, IEEE Intelligent Systems, 2014.
- J. Guha, N. Daswani, and R. Jain, “Machine Learning for Online Advertising”, ACM SIGKDD Explorations Newsletter, 2012.
- J. Zhang, Y. Zhao, and Y. Zhou, “Machine Learning in Demand Forecasting for E-commerce”, Proceedings of the 3rd International Conference on Intelligent Computing and Cognitive Informatics, 2018.